Hybrid AI Model CausVid Generates High-Quality Videos in Seconds
Share
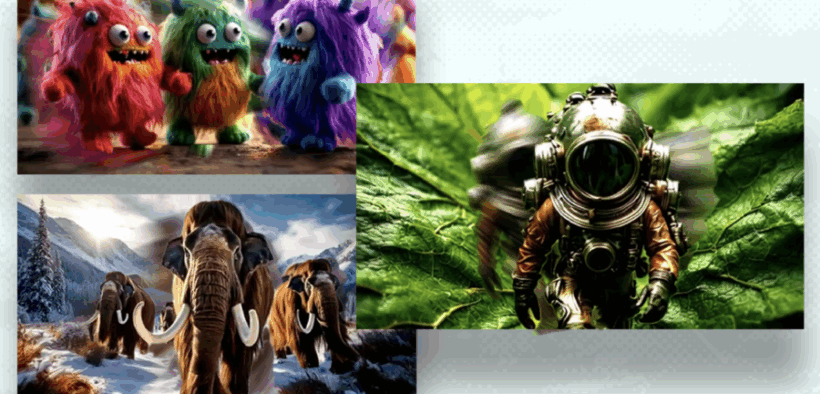
A team of researchers from MIT’s Computer Science and Artificial Intelligence Laboratory (CSAIL) and Adobe Research has unveiled a groundbreaking hybrid AI model that dramatically accelerates video generation while preserving visual fidelity. The model, dubbed CausVid, merges two powerful AI approaches—diffusion models and autoregressive systems—to create smooth, high-resolution videos from text prompts in just seconds.
A Smarter, Faster Way to Animate
Unlike traditional autoregressive models that generate videos one frame at a time—often leading to jittery visuals and degraded quality—diffusion models like OpenAI’s SORA or Google’s VEO 2 generate entire video sequences at once. While this produces photorealistic results, it’s computationally intensive and ill-suited for interactive editing or real-time generation.
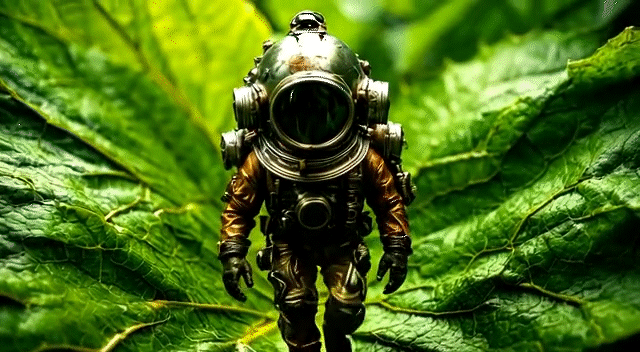
CausVid bridges that gap. The system uses a pre-trained diffusion model as a “teacher” to guide a lightweight, frame-by-frame “student” model. This hybrid approach allows for rapid video creation with high consistency and quality—enabling dynamic features like real-time prompt updates, scene extensions, or video alterations mid-generation.
“CausVid combines the long-range coherence of diffusion models with the responsiveness of autoregressive systems,” said Tianwei Yin SM ’25, PhD ’25, co-lead author of the paper. “It’s like teaching a fast student to think like a wise teacher.”
From Concept to Scene in Seconds
CausVid can generate imaginative and artistic visuals from simple prompts—like a paper airplane transforming into a swan or a child splashing in a puddle—and seamlessly extend or edit the results on the fly. For instance, a user could start with “a man crossing the street,” then update the prompt mid-generation to “he writes in his notebook on the opposite sidewalk.”
In benchmark tests, CausVid outperformed leading video generation systems, including OpenSORA and MovieGen, producing 10-second high-res clips up to 100 times faster while maintaining greater visual stability and coherence. It also excelled with 30-second videos, paving the way for longer sequences—potentially hours in length or even continuous streams.
In a user preference study, participants favored clips made by CausVid’s student model over those from the more computationally expensive diffusion teacher model.
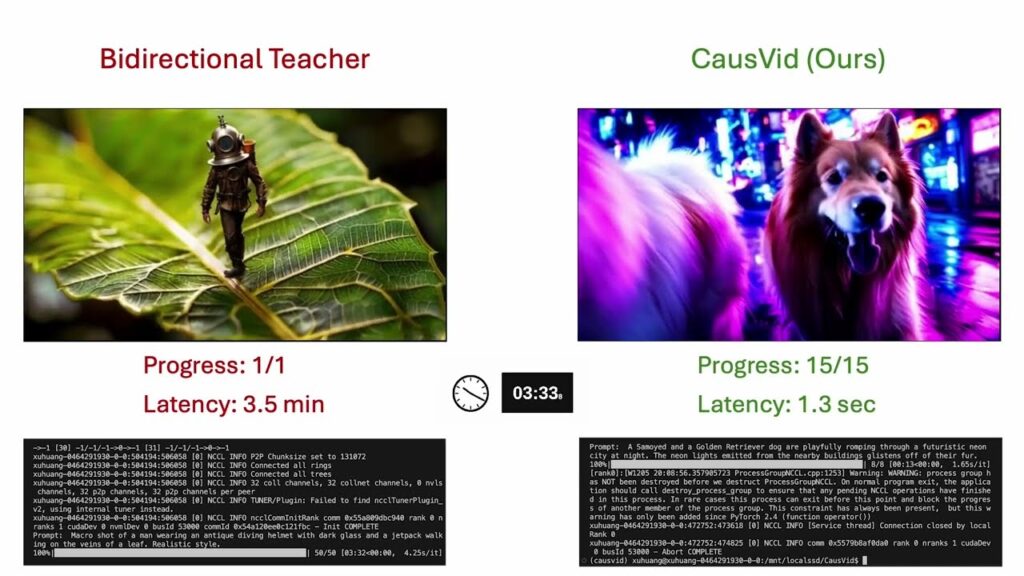
Real-World Applications: From Gaming to Robotics
Beyond creative content generation, the model opens up possibilities in several industries:
- Language translation: Generating synced video content from translated audio streams.
- Video games: Rapid scene rendering for immersive, reactive gameplay.
- Robotics: Generating training simulations to teach AI systems physical tasks.
- Livestreaming: Smoother, real-time enhancements with lower latency and reduced energy use.
Because CausVid’s architecture can be fine-tuned on domain-specific datasets, its creators believe it could soon deliver even higher-quality content tailored to specialized fields.
A New Benchmark for Speed and Quality
When tested on more than 900 prompts using a standard text-to-video dataset, CausVid achieved an overall score of 84.27, the highest among comparable models. It led in categories such as visual quality and realistic human motion, surpassing state-of-the-art systems like Gen-3 and Vchitect.
“Diffusion models are far slower than LLMs or image generators,” said Jun-Yan Zhu, an assistant professor at Carnegie Mellon University who was not involved in the project. “This new hybrid approach changes the game—it enables faster streaming, interactive applications, and even reduces the environmental impact.”
What’s Next?
The researchers aim to make video generation even more efficient by shrinking the causal model and optimizing training on focused datasets. That could bring near-instant generation and unlock new use cases in real-time simulation, animation, and digital communication.
CausVid will be formally presented at the Conference on Computer Vision and Pattern Recognition (CVPR) in June. The research was supported by the Amazon Science Hub, Adobe, Google, the U.S. Air Force, and other institutions.