Ant Group Taps Chinese Chips to Train AI Models, Cut Costs
Share
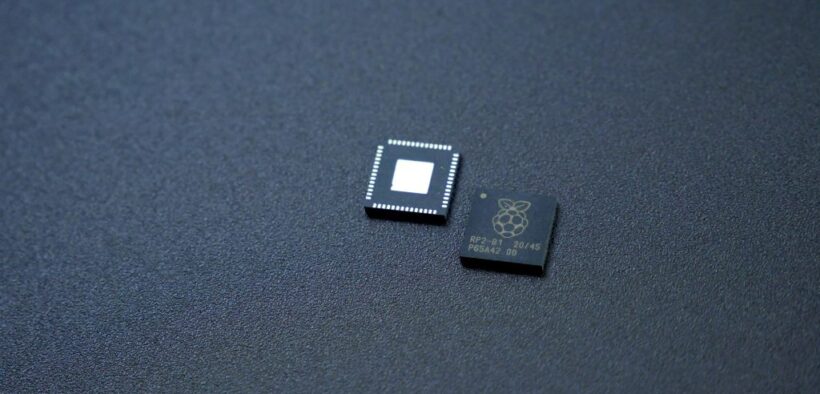
Ant Group is increasingly relying on Chinese-made semiconductors to train large-scale AI models, a strategic move aimed at lowering costs and reducing dependence on restricted U.S. technology, according to sources familiar with the company’s operations.
The Alibaba affiliate has reportedly used domestic chips—including those linked to Alibaba and Huawei Technologies—to train large language models using the Mixture of Experts (MoE) approach. Sources say the results were comparable to models trained on Nvidia’s H800 GPUs. While Nvidia chips remain in use at Ant, the company is gradually shifting toward alternatives from AMD and Chinese semiconductor makers for its latest AI projects.
This development highlights Ant’s growing role in China’s broader AI race with the United States, as domestic tech firms seek cost-effective workarounds to U.S. export controls. These restrictions limit access to advanced chips such as Nvidia’s H800—a powerful, though not top-tier, GPU still widely used in China.
In a newly published research paper, Ant claims its MoE-trained models achieved performance levels that, in some cases, surpassed those developed by Meta. Bloomberg, which first reported the story, noted that the findings have not been independently verified. If confirmed, the results could mark a significant advance in China’s push to reduce its reliance on foreign hardware for AI development.
MoE: A Cost-Cutting Strategy
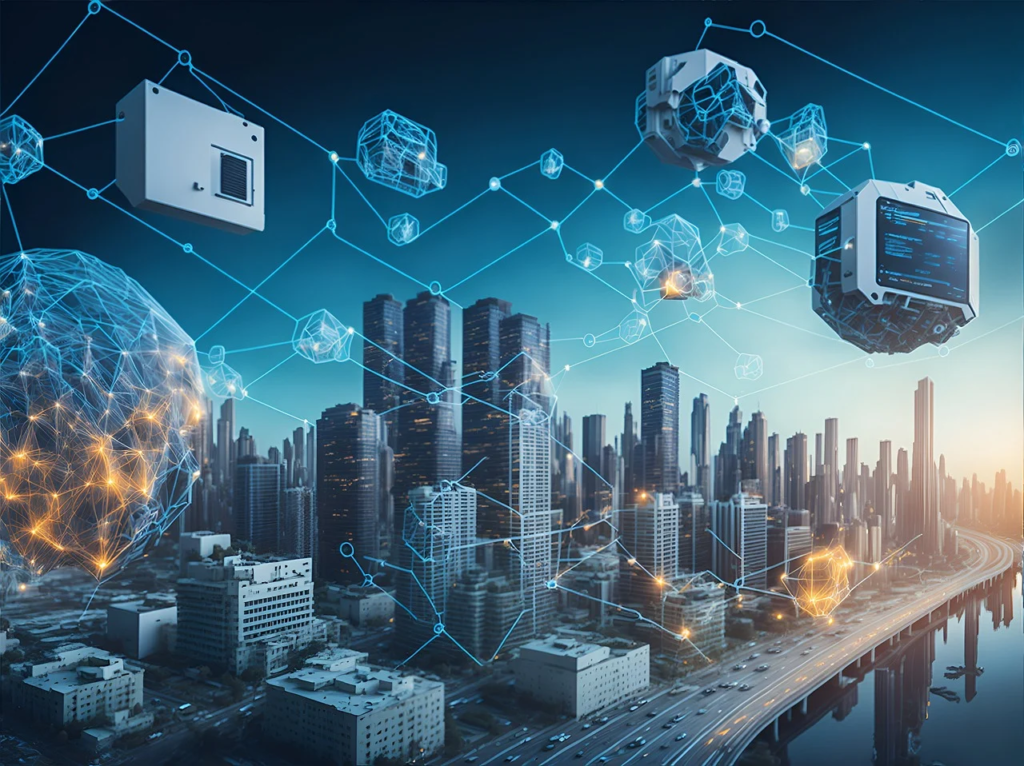
The MoE architecture divides tasks into smaller components that are distributed across different “expert” modules. The technique has drawn growing interest from major AI players like Google and DeepSeek, a Hangzhou-based startup. Comparable to assembling a team of specialists, MoE enables more efficient model training—especially critical as high-end GPUs become cost-prohibitive for many firms.
Ant’s research aimed to prove that effective AI training doesn’t require access to premium hardware. The paper is explicitly subtitled “Scaling Models without premium GPUs.”
According to the study, training a trillion tokens using conventional top-tier GPUs cost about 6.35 million yuan (approximately $880,000). Ant’s optimized approach brought that figure down to around 5.1 million yuan by using lower-spec chips—an 18% reduction in training costs.
This cost-conscious strategy contrasts sharply with Nvidia’s vision. CEO Jensen Huang has said that demand for computing power will continue to soar, even as models become more efficient. He argues that businesses will prioritize performance and scale over cost savings—positioning Nvidia to continue building ever-more powerful chips with higher core counts and memory capacity.
From Research to Real-World AI Deployment
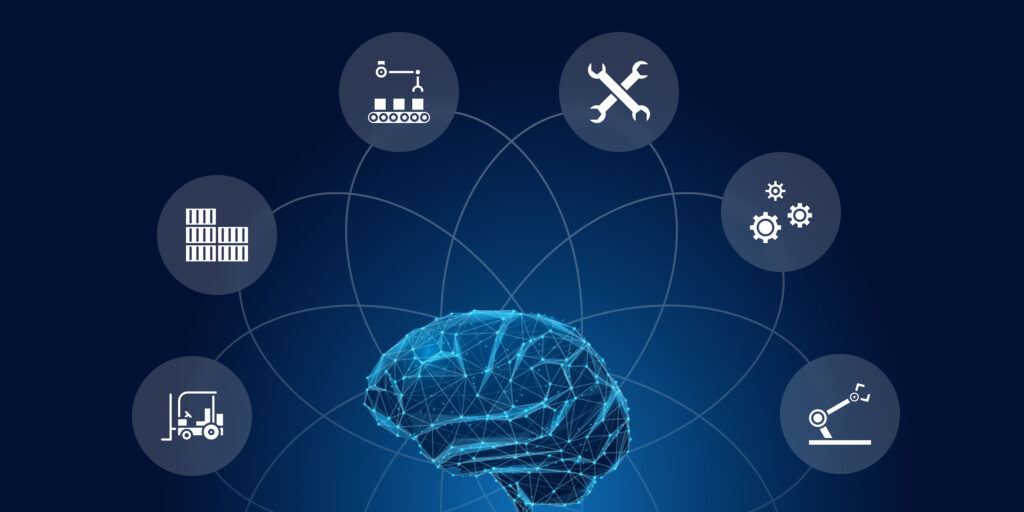
Ant’s open-source models, Ling-Lite (16.8 billion parameters) and Ling-Plus (290 billion parameters), are intended for use in industries like healthcare and finance. Earlier this year, the company acquired Haodf.com, a Chinese online medical platform, in a bid to expand its AI-driven healthcare offerings. Ant also runs services such as the AI virtual assistant Zhixiaobao and the financial advice platform Maxiaocai.
As Robin Yu, CTO of Beijing-based Shengshang Tech, put it:
“If you find one point of attack to beat the world’s best kung fu master, you can still say you beat them—which is why real-world application is important.”
Despite its progress, Ant acknowledges the technical challenges involved. The paper notes that even small tweaks to model architecture or hardware can lead to unstable performance and sudden spikes in error rates—highlighting the fragile balance of large-scale AI development.
Still, Ant’s success with MoE on domestic hardware may signal a pivotal moment in China’s ongoing effort to localize AI infrastructure—potentially reshaping the global dynamics of AI advancement.